Modan Tailleur
I am a PhD student specializing in the sound field with a focus on deep learning. Through my expertise in research and my experiences as a sound engineer, I aim to contribute meaningfully to the advances of the audio research field. Here, you'll find details about my academic journey, skills, and projects. Thank you for taking the time to visit !
About
- Languages: French (native), English (fluent), Italian (basic), German (basic), Japanese (basic)
- Programming Languages: Python, C, C++, HTML/CSS, Java, JavaScript, Bash, VBA
- Databases: MySQL, PostgreSQL
- Libraries: PyTorch, TensorFlow, NumPy, Pandas
Experience
Sound source detection for the sensitive mapping of urban sound environments.
👉 Deep Learning: classification, generative models
👉 Statistical analysis, experimental design, auditory perception, cognitive psychology
👉 Acoustic scene analysis, audio signal processing, programming (Python)
Skills: Computer Science, Statistics, Python, Deep Learning, Acoustics, Cartography
Teaching students from the 1st to the 3rd cycle
👉 Deep Learning
👉 algorithms and programming
👉 signal processing
👉 SQL database
Skills: Pedagogy, Python, C++, SQL, Deep Learning, Acoustics
Freelance sound engineer working for radio, music and cinema
👉 Sound mixing and sound editing for the cinema industry, for music, and for sound fictions
👉 Direction of a radio program for Prun' radio
Publications
Workshops
Slow or fast third-octave bands representations (with a frame resp. every 1-s and 125-ms) have been a de facto standard for urban acoustics, used for example in long-term monitoring applications. It has the advantages of requiring few storage capabilities and of preserving privacy. As most audio classification algorithms take Mel spectral representations with very fast time weighting (ex. 10- ms) as input, very few studies have tackled classification tasks using other kinds of spectral representations of audio such as slow or fast third-octave spectra.
In this paper, we present a convolutional neural network ar- chitecture for transcoding fast third-octave spectrograms into Mel spectrograms, so that it could be used as input for robust pre-trained models such as YAMNet or PANN models. Compared to training a model that would take fast third-octave spectrograms as input, this approach is more effective and requires less training effort. Even if a fast third-octave spectrogram is less precise both on time and frequency dimensions, experiments show that the proposed method still allows for classification accuracy of 62.4% on UrbanSound8k and 0.44 macro AUPRC on SONYC-UST.
Urban noise maps and noise visualizations traditionally provide macroscopic representations of noise levels across cities. However, those representations fail at accurately gauging the sound perception associated with these sound environments, as perception highly depends on the sound sources involved. This paper aims at analyzing the need for the representations of sound sources, by identifying the urban stakeholders for whom such representations are assumed to be of importance. Through spoken interviews with various urban stakeholders, we have gained insight into current practices, the strengths and weaknesses of existing tools and the relevance of incorporating sound sources into existing urban sound environment representations. Three distinct use of sound source representations emerged in this study: 1) noise-related complaints for industrials and specialized citizens, 2) soundscape quality assessment for citizens, and 3) guidance for urban planners. Findings also reveal diverse perspectives for the use of visualizations, which should use indicators adapted to the target audience, and enable data accessibility.
This paper explores whether considering alternative domain-specific embeddings to calculate the Fréchet Audio Distance (FAD) metric can help the FAD to correlate better with perceptual ratings of environmental sounds. We used embeddings from VGGish, PANNs, MS-CLAP, L-CLAP, and MERT, which are tailored for either music or environmental sound evaluation. The FAD scores were calculated for sounds from the DCASE 2023 Task 7 dataset. Using perceptual data from the same task, we find that PANNs-WGM-LogMel produces the best correlation between FAD scores and perceptual ratings of both audio quality and perceived fit with a Spearman correlation higher than 0.5. We also find that music-specific embeddings resulted in significantly lower results. Interestingly, VGGish, the embedding used for the original Fréchet calculation, yielded a correlation below 0.1. These results underscore the critical importance of the choice of embedding for the FAD metric design.
In this paper, we introduce the Extreme Metal Vocals Dataset, which comprises a collection of recordings of extreme vocal techniques performed within the realm of heavy metal music. The dataset consists of 760 audio excerpts of 1 second to 30 seconds long, totaling about 100 min of audio material, roughly composed of 60 minutes of distorted voices and 40 minutes of clear voice recordings. These vocal recordings are from 27 different singers and are provided without accompanying musical instruments or post-processing effects. The distortion taxonomy within this dataset encompasses four distinct distortion techniques and three vocal effects, all performed in different pitch ranges. Performance of a state-of-the-art deep learning model is evaluated for two different classification tasks related to vocal techniques, demonstrating the potential of this resource for the audio processing community.
Journals
The exploration of the soundscape relies strongly on the characterization of the sound sources in the sound environment. Novel sound source classifiers, called pre-trained audio neural networks (PANNs), are capable of predicting the presence of more than 500 diverse sound sources. Nevertheless, PANNs models use fine Mel spectro-temporal representations as input, whereas sensors of an urban noise monitoring network often record fast third-octaves data, which have significantly lower spectro-temporal resolution. In a previous study, we developed a transcoder to transform fast third-octaves into the fine Mel spectro-temporal representation used as input of PANNs. In this paper, we demonstrate that employing PANNs with fast third-octaves data, processed through this transcoder, does not strongly degrade the classifier's performance in predicting the perceived time of presence of sound sources. Through a qualitative analysis of a large-scale fast third-octave dataset, we also illustrate the potential of this tool in opening new perspectives and applications for monitoring the soundscapes of cities.
Projects
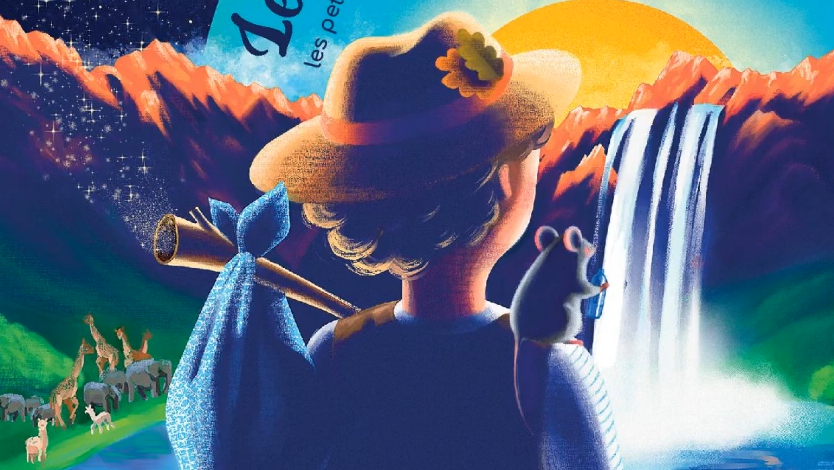
A musical story written, composed, and performed by Mikael Tailleur, directed by Modan Tailleur.
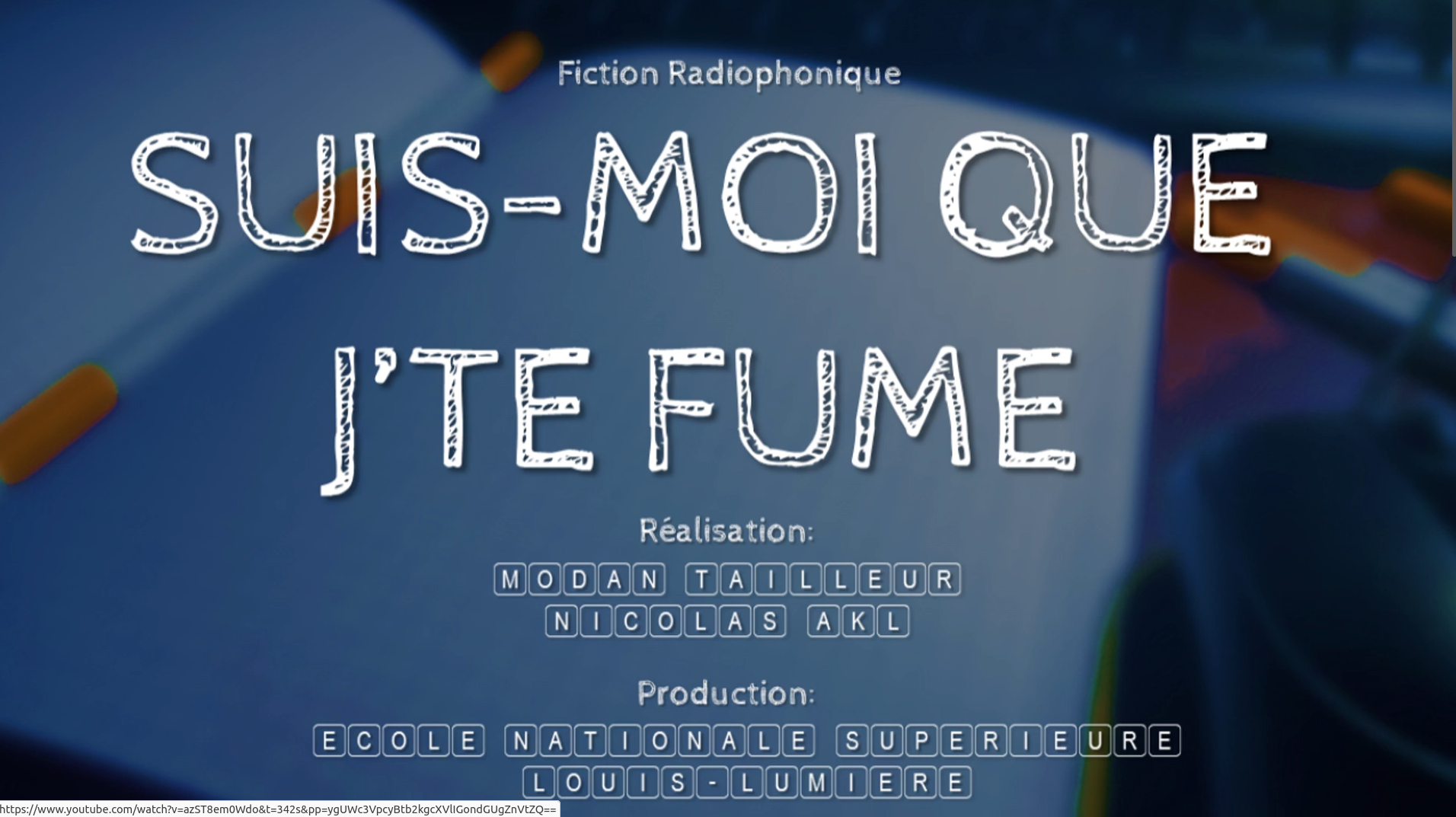
A sound fiction written and directed by Modan Tailleur and Nicolas Akl.
Despite Fabien's hostile attitude during his job interview, Corentin was eventually accepted for an internship at Cintroflex.
While not the professional experience of his dreams, this internship still allows him to spend some more time with his grandmother. Unfortunately for him, Fabien still has a few surprises in store.
Education
Nantes, France
Degree: PhD in computer science
- Deep Learning
- Acoustics
- Cartography
Relevant Courseworks:
Châlons-en-Champagne , France
Degree: Generalist Engineer Diploma (master)
- Mechanics
- Database Management Systems
- Operating Systems
- Electronics
Relevant Courseworks:
Bari , Italy
Degree: Master in mechanical engineering
- Mechanics applied to aeronautics
- Management of innovation
- Labor Law
Relevant Courseworks: